When I first used Dall-E in January 2021, I input some text, I think it was a resin chair inspired by a cactus (I had Gaetano Pesce on my mind). It gave me an output that genuinely rocked me. I was testing this mental model that many people have for image generation that it’s basically just collaging existing images together and I realised fast that this was a very bad mental model. The first output blew my mind because it was so unexpected; It created a lot of detail within the image that I didn’t imagine when I wrote the prompt, which I could never find with a search engine. It had interpreted my prompt in a what I perceived as a creative way. I spent that day testing the limits, looking for more unexpected outputs and using this tool creatively. Over the course of the next year I continued to generate images, but at some point during this year my thinking shifted. I started using generative AI to produce something that I had a very clear image of in my mind, and stopped looking for those unexpected creative outputs. AI became a fast route to passable outputs, and gradually I let my standards slip, accepting more boring images with an AIsthetic that weren’t actually that close to my vision. This was a huge mistake and it’s a mistake that’s flooding the internet and our culture with homogenous AI imagery. Instead of using AI to make familiar work, only more of it and faster than ever, we should be using AI to make better, more creative work.
This is important not just for those using generative AI systems as a tool but also for those developing them. Currently in the development of AI, the emphasis seems to be on executing jobs that humans are already doing pretty effectively. Some of the biggest current applications are customer support, financial services and marketing - there’s room for creativity in these applications, but it’s hard to imagine creativity is their priority. To me the most exciting applications are those with problems that need creative problem solving - whether in the industries we think of as ‘creative’ or at the forefront of research for other industries like drug discovery or climate modelling. I use image generation tools frequently and they provide a clear example of how we are optimising for consistency instead of creativity. Image generation models undergo training via reinforcement learning with human feedback (RLHF) in order to try to align AI with aesthetics valued by humans. But in order to do that we have to define a ‘desirable aesthetic’ and then reinforce these strict beauty standards, narrowing the scope of generative outputs in the process. If you’ve started to notice a homogenous AIsthetic proliferating on the web, this is why.
To be clear, I’m not saying we should replace all our creatives and artists with AI agents, in fact the opposite; I’m saying more artists should use AI as a new tool to make more creative work. The biggest mistake here would be for clients to cut the artists out and generate media directly, because suddenly they can generate passable, cheap interior design photos, fashion shoot imagery, product design shots, [insert visual content here]. Sadly this full scale automation is starting to happen already, and it does not result in creative outputs. AI doesn’t generate creative outputs by default, but it can augment creativity in the right hands.
I trained and worked in architecture, and I’ve thought a lot about how generative AI fits into this profession. When I talk to friends that continued to work as architects (or many other creative professions) there’s usually a similar set of questions : ‘can i use this to do rendering more quickly?’ ‘will this expedite material specification for me?’. (the answer is yes, but that’s not the takeaway). This is also the way I used to think about it - ‘how does this new tool fit into the design framework I know and love?’ Or more cynically - ‘can I use this to automate the boring task that is part of my job so I can focus on the fun stuff’. But it’s much more taboo to use a computer program for the actual idea creation. The core value of any creative profession is in coming up with new, good ideas, and the notion that generative AI might be able to help with this appears as an existential threat. But accepting AI as a creative collaborator could be vital to expanding our creative potential, so I want to explain why I think of AI as creative.
Generative AI is creative
Creativity can be a vague and misused word, so let me first say that by creative I mean something that is novel and holds value.
With that criteria in mind, hopefully the idea that genAI can be creative should be intuitive for those that have spent a lot of time using generative softwares, particularly with image generation [side note]. But it’s counter-intuitive from a technical perspective: How can an algorithm produce anything new, when it’s designed to emulate or represent/compress an existing dataset?
There’s a popular idea that there are three kinds of creativity : explorative, combinative, and transformative. I’m going to lay out each of these modes of creativity and suggest that generative AI is good at the first two, and bad at the last.
Side note
Although I think these ideas are equally relevant for language as for image, I’ll mostly reference image generation here, as it’s been my focus for the last few years. I also think the opportunities of creative output are higher with images. I ran a quick experiment testing this, asking subjects to perform the same task with image and text generators, and asked them to rank the outcomes according to how ‘unexpected’ they were. It was a pretty basic sense-check (with a sample size of 5) but all of them told me that the image generation results were more unexpected than text generation. I have a few theories for why this is, one of which is that people are more likely to continue being taught to write throughout their lives than to create imagery. As a result when they take to midjourney with a prompt they have a less concrete vision of what they want than those that take to chatgpt with a prompt. This results in image generation requests that place less constraints on the model and leave more room for creativity. More on this in another post.
Exploratory creativity
Exploratory creativity is about discovering the peripherals of an established system or domain. It involves working within a framework but exploring its limits and possibilities in-depth. This is perhaps the most obvious creative application for AI. Once you have defined the scope of an aesthetic style, you can use AI to fully explore that style at a high speed, rattling through generations until arriving at something of value. Maybe defining the style and design system is the tricky part here, but there are various ways to loosely fine-tune or guide an image generation to a particular aesthetic. In this way you can explore motifs and how they apply to a wide variety of subject matter.
This can be used to go deeper than purely aesthetic style, and comes closer to something like defining a gestalt, but I’m using aesthetic style as an easily recognisable example. In reality, well defined parameters for exploration here could include the subject, composition, aesthetic and emotion amongst really any other image characteristic you could imagine.
example 1 - “The intersection of nature, architecture, and technology has inspired my work in recent years. I’ve been using different techniques where CGI it’s been the primary creative tool for digital and physical outputs.
In the meantime, I found myself creating and training an AI model, using the work I’ve created the last 10 years, and exploring how it could be added to my practice. Bringing new perspectives and possibilities.
These are images from one of the concepts I created, based a new path I’m exploring this year in the studio.” - @sixnfive
example 2 - An often quoted example (because it’s a good one) of creative AI is the famous Go match between Korean master Lee Sedol and Deepmind's AlphaGo. In move 37 of the second game, AlphaGo made a winning move that defied 4000 years of precedent. This is a perfect example of why AI is excellent at explorative creativity. When given a clearly defined domain, AlphaGo was able to explore new depths of the domain, leveraging it’s capacity to calculate through games, and cross reference them against it’s own value system.
In the first image generation example, the model doesn’t know what is of value to the user; The task of curating what’s valuable falls to the artist. Still, the image generator has made something novel and of value, whether or not it could recognise it, so I attribute it with creativity. (I’m saying AI can be a valuable creative collaborator, not that it will replace human creatives.)
Creative combination
This kind of creativity involves finding new ideas through the combination of seemingly unrelated fields or elements. In my view this is where generative AI really excels.
In machine learning there is a term used to describe how a model internally represents data called ‘latent space’. This is the multidimensional space within which, all the data that the model has been trained on, can be represented. The beauty of latent space is that it’s the ultimate leveller. You can combine two words that may be entirely semantically different, which never appear together in a single piece of training data, yet the model can represent them both within this space, finding connections between them. I doubt there is an image in midjourney’s training set which is labelled with both ‘balenciaga’ and ‘the pope’ for example.
example 1 - pope in balenciaga drip
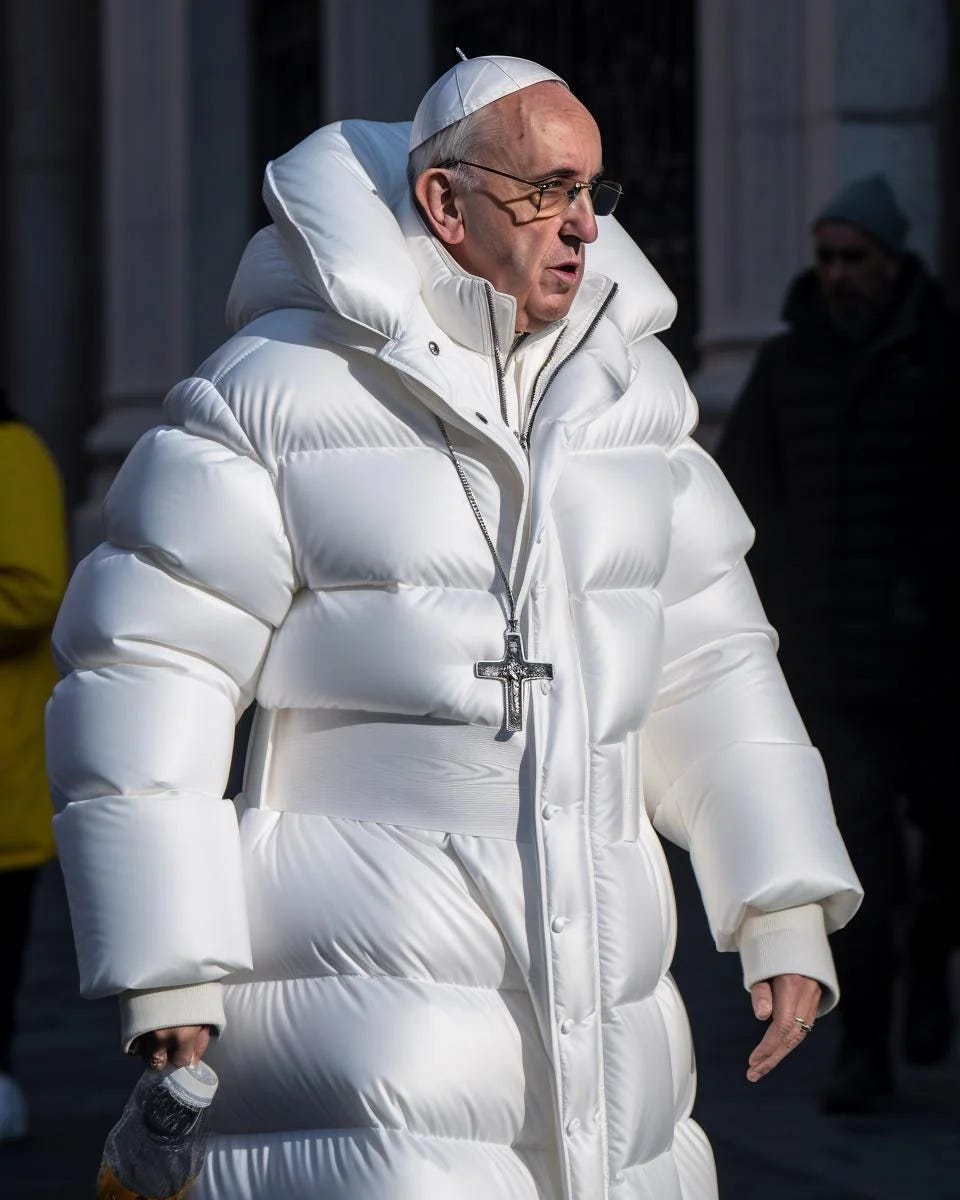
This is a pretty iconic image from the early days of Midjourney which did the rounds on reddit. It’s great, it works so well that you almost don’t realise that this isn’t the Pope’s everyday winter outfit. Clearly the idea to bring these two disparate worlds of Balenciaga and the Catholic Church together belongs to the user. But the diffusion model brings the two ideas together with creative details that brings the combination to life. The distinctive overly puffy puffer-jacket is pinned in by the wide papal fascia waist band. It has a sequence of small buttons that you would find on a cassock but never on a puffer jacket, but which don’t feel out of place. The pectoral cross seems extra bling but maybe it is just like that. There’s a host of small details like that, which make the image convincing, down to quality of the photograph, with its low depth of field and high zoom making it look like a paparazzi snap.
example 2 - An image I generated that will be exhibited at IAMA, San Francisco in October, 2024
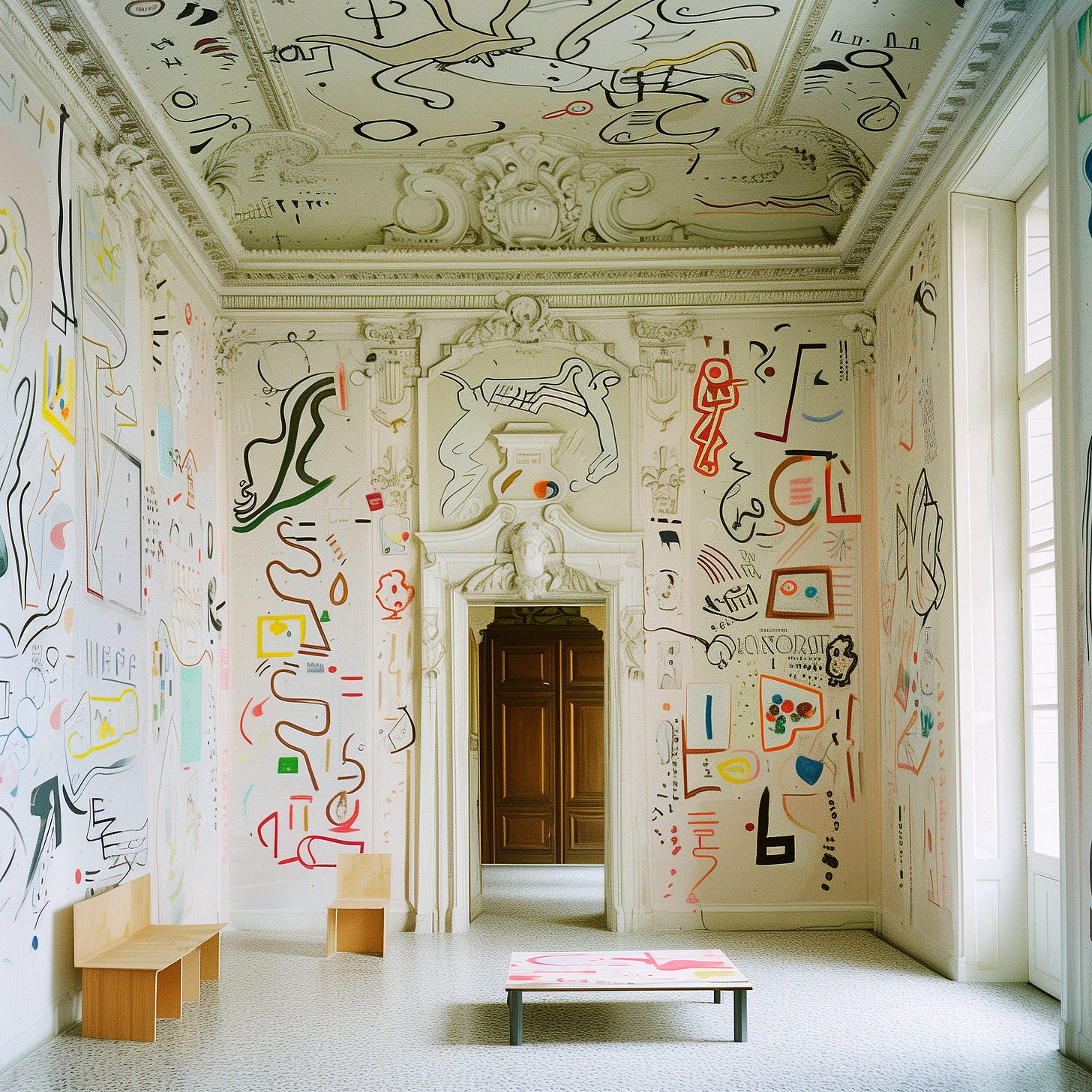
I saw some images of Basquiat’s studio a couple of months ago with paintings drawn haphazardly across the walls. It was a warehouse studio and the artwork didn’t look too out of place, but I wanted to imagine this kind of spontaneous work on the walls of the careful rules-based neoclassical architecture. At the same time I wanted to include Joan Miro and Keith Haring, and give some complexity to the space similarly to Ricardo Bofill’s architecture. I had these ideas and inspirations, and the notion that they might come together in an interesting way, but not really an idea of their collective embodiment. That’s where AI is so powerful - it can pull details from all of these domains into coherent imagery that contains creativity and further sparks new ideas for the user. It’s not like they created perfect imagery. I sifted through hundreds of outputs, regenerating details and photoshopping and post processing until I was happy with the results. But I attribute some of the creativity of these images to the algorithm.
There’s an important discussion about ownership and artist accreditation with this example, and I’m including the prompt to be clear about which artists that I have directly prompted for, but I also realise this doesn’t cut it, and am planning to write more about how we could do better at attribution in future.
Creative transformation
I find this idea of transformational creativity a little hard to buy into if I’m being honest : Creating something that is so different from the existing domain, that it defines a new paradigm. It sounds too much like original ideas come entirely from nothing… just an individuals genius, rather than a combination of inspirations contributing to emergent ideas. More likely I think, is that an individual is inspired by a medium or domain so unrelated to the one that’s being targeted, that it cannot be traced back to that inspiration and is called transformational creativity. It’s the subconscious application of entirely different domains (like being inspired to draw by something you listened to), in other words - a high level form of combinative creativity. So if diffusion models are great at combinational creativity then they should be great at transformational creativity too? But the problem is that these image generation models only trained on the domain of imagery. Even more than that they’re only trained on the correlation of image with language (most of the time) not the correlation of images with music, emotional response, smell or anything else a human might associate an image with.
The current image generation models we’re working with in fact have a stringent domain defined by images that are a certain number of pixels wide and tall, that each have an RGB value. There are multimodal models, but they’re still just dealing with a very limited form of training data in comparison with the human experience. If we can think of humans as products of their experiences in the same way as AI models, then their domain is so complex that it becomes intangible and magical. Human experience includes the visual, textual, audible, social and contextual and the interactive amongst whatever else we don’t understand. Maybe these are the things that lead to insights so unquantifiable that we call them transformational creativity, or maybe it’s some entirely different human mechanism.
example - Les Demoiselles d’Avignon
An often cited example of transformative creativity in the art world is the development of cubism. The breakthrough for this new style is usually attributed to the work of Georges Braque and Pablo Picasso and particularly to Les Demoiselles d'Avignon. It’s a good example of an aesthetic that drastically breaks tradition from the previous zeitgeist of impressionism and realism. It’s hard to trace back the aesthetic roots to any one influence or inspiration, and in reality the work is likely the result of Picasso’s diverse lived experience. Influences might have as easily included preceding visual artworks like Iberian sculptures or African masks and statuettes as Stavinsky’s ballet 'The Rite of Spring’.
So what?
Lets use AI to be more creative not to make more boring stuff faster. AI is not just a tool for automation. It’s not just a way of collaging existing images together, it’s a new creative tool, that will help you to improve the quality of our work. We can use it as a collaborator to have more diverse ideas, to formalise an abstract idea or just to break through a creative block.
If it keeps going the way it’s going, the internet’s imagery could be 90% generative within the next few years. If we continue to develop and use generative AI as a tool for efficiency instead of a creative agent, then that imagery will become more and more homogenous. As artists we should be pushing the limits of generative AI, looking for unexpected outputs and pushing back against the narrowing scope of emergent AIsthetics. As builders and developers we should stop narrowing the scope and prioritise making a larger search space available to users.
END
That’s all I’ve got for now but there are lots of related bits in the works so sign up for the newsletter if you’re interested :)
Huge thanks to Liz Lee, Arthur Laidlaw and Tom Ough for their thoughtful comments on this! Rogue opinions and literary mistakes are all my own despite their best efforts.
If anyone out there is developing AI systems and actively optimising for creativity, I’d love to chat about it, so please get in touch!